Emotion AI: 3 Experts on the Possibilities and Risks
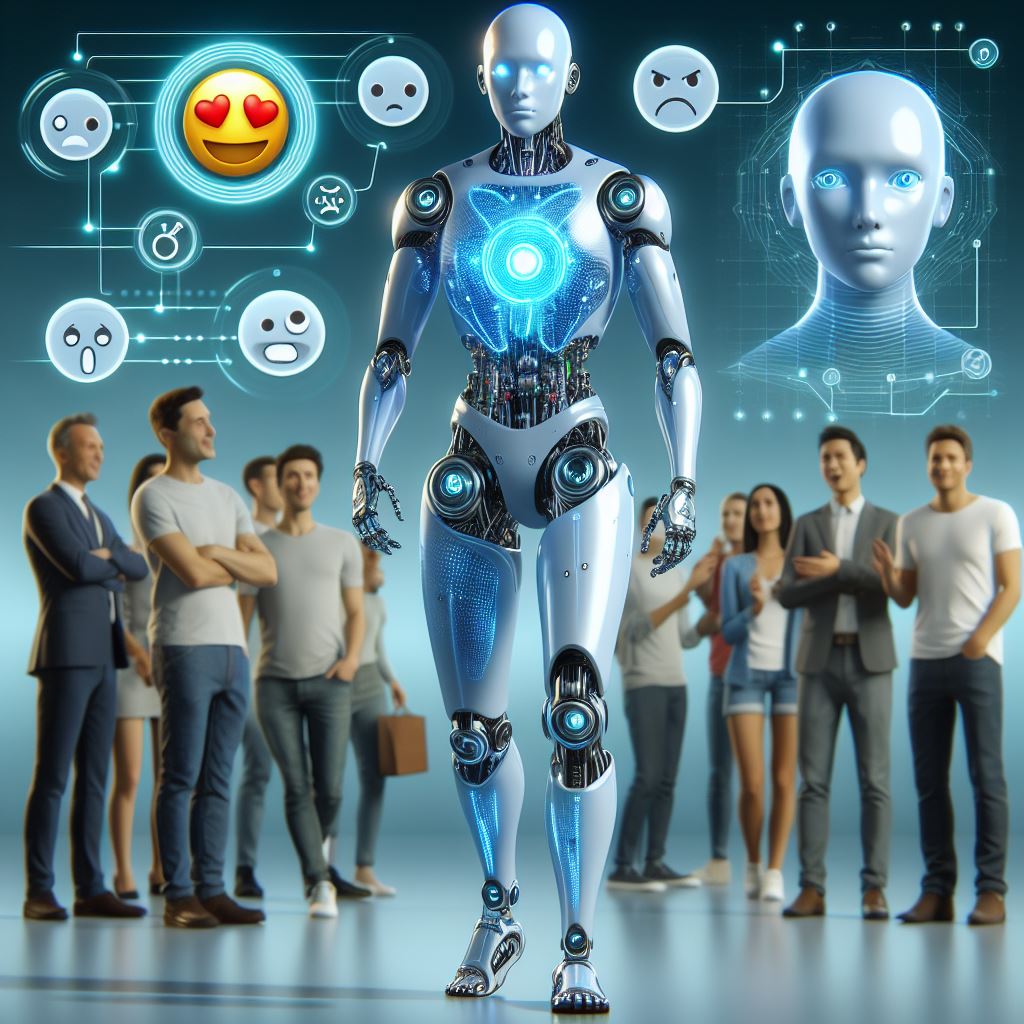
Emotion AI is a fantastic piece of technology that helps computers understand and respond to how people feel. It’s like when your phone suggests emojis based on what you type—but way more advanced. This tech uses language analysis, checking the tone of your voice and even studying your face to figure out your emotions from texts, sounds, and videos.
You’ll find Emotion AI in many places without even realizing it. For example, companies use it to determine how people feel about their products by reading reviews or suggesting things they might like. It’s also used in finance to predict stock market trends.
And it’s not stopping there. In the future, Emotion AI could help doctors diagnose depression, spot when someone is trying to trick an insurance company, help teachers understand if students are getting their lessons, or make sure drivers focus correctly. The interest in this technology is growing fast, and it’s expected to become even more popular and valuable by 2027.
Advantages of Emotion AI
Emotion AI is super helpful for many jobs – from marketing folks to engineers to people who make ads and design stuff. It can give them vital clues about what people like or don’t like without asking them directly.
This cool tech makes it easier and quicker to see what people think about new products or ads by understanding their feelings and reactions. So, you’re designing a new app or an advertisement. In that case, you can use Emotion AI to see if people are happy, bored, or not like it, saving time and money.
Because Emotion AI is so good at picking up on how we move and feel, the things made with it are more likely to be something we enjoy using or find really useful because they fit better with what we need and how we feel.
Disadvantages of Emotion AI
Even though Emotion AI can do many cool things, it could be better. Figuring out what someone is feeling can be really tricky, and using this technology in serious situations could cause problems.
For example, a company once tried to use Emotion AI to decide if someone would be good for a job based on their facial expressions and how they talked, which isn’t a great way to judge someone’s ability to work.
Like any other tech, Emotion AI can make mistakes and might be only for everyone. For Emotion AI to watch and analyze how we feel, we have to say it’s okay, which could make some people worried about their privacy.
Types of Emotion AI
Emotion AI comes in three excellent types, each looking at different ways we express ourselves:
- Text Emotion AI: This type looks at what we write, like online comments or news articles, to determine if what’s being said is happy primarily or sad. Imagine scanning many tweets to see if people feel good or bad about something.
- Voice Emotion AI: This focuses on how we sound when we talk. It’s used a lot in places like customer service to understand the tone of the conversation and what’s being talked about, helping to see if customers are happy or frustrated.
- Video and Multimodal Emotion AI: This one is super advanced. It checks out videos to catch all sorts of signals, from where you’re looking to how you’re moving. It’s like assembling the puzzle of someone’s emotions by watching them.
To get the scoop on how Emotion AI really works, we listened to four intelligent people who know a lot about it: Seth Grimes, who helps others understand natural language processing; Ranal Gujral, who’s the boss of a company called Behavior Signals; Skyler Place, who knows a ton about behavioural science at Cogito; and Daniel McDuff, who used to research AI at Microsoft. They shared some incredible insights about these three types of emotional AI, making it easier to get why it’s so fascinating.
Text Emotion AI: NLP and Sentiment Analysis
Sentiment analysis is like using a computer to figure out if what people write or say is happy, sad, or something in between. It’s a way for companies to see what people think about their stuff by looking at the comments and reviews people leave online.
HOW DOES TEXT EMOTION AI WORK?

Seth Grimes talks about a smart way to teach computers using transfer learning. Imagine a big, intelligent computer brain already trained on many things. But to make it perfect for what you specifically need, add some extra learning at the end, using your own information. This helps the computer get really good at understanding exactly what you want it to.
For example, a company like Syler Placeny might have a computer program that knows all about hotels, such as the rooms, service, and restaurants. However, the unique things about Hilton Hotels might have yet to be discovered, like their special rewards program, Hilton Honors. In the past, people taught computer skills by hand. But now, they use transfer learning to teach the computer the unique things about Hilton or anything else more easily and quickly, making it super bright about that particular topic.
HOW DOES EMOTION AI DEAL WITH COMPLEXITY?
Grimes: Sentiment analysis is a tech tool that tries to determine whether people’s words are positive or negative, but it’s a bit tricky and not always right. For example, there’s a story about using a picture of Kobe Bryant smiling. Since Kobe Bryant passed away in a sad accident, a smiling photo might make you feel happy because of the smile or sad because of the memory. It shows how complex and personal our feelings can be.
A company called Clarabridge is working on improving sentiment analysis. They’ve gone beyond just saying things are good or bad. Now, they can identify specific emotions like happiness, sadness, or anger. They’ve also created a way to show how strong these feelings are, from really mild to super strong. This helps understand emotions in a more detailed way instead of just saying something is good or bad.
HOW ACCURATE IS TEXT EMOTION AI?
Grimes: When we talk about being accurate in understanding people’s opinions, there are two main things to consider: precision and recall. Precision is like aiming at a target. If you ask whether someone liked or didn’t like something, like a hotel stay, that’s too broad. For example, if someone says they had a great time on their vacation, what exactly did they like? The room, the staff, the food, or the location? That’s where “aspect-based sentiment analysis” comes in, a fancy way of looking at specific parts of a review. It helps you understand exactly what people liked or didn’t like.
A while back, companies began making their analysis more detailed. They started making different tools for different things – one for restaurants, another for hotels, and another for gadgets.
For instance, saying a phone is thin might be good, but saying hotel sheets are thin definitely isn’t. So, it’s essential for these tools to know the difference and to be trained in specific industries to be really useful.
HOW CAN TEXT EMOTION AI BE ABUSED?
Grimes shared a really concerning idea about how information on how we feel could be used in ways we might not expect. Imagine if, based on what someone writes online or to a helpline, it was possible to guess they might be thinking about hurting themselves.
What if their health insurance company found out and used that info? Or imagine a car insurance company could tell someone was driving while angry just by looking at their facial expressions and deciding to charge them more, thinking they might be more likely to crash.
Whether these uses of emotional data are wrong depends on how you see things. Still, they’re not what people think will happen when they express their feelings. They could be seen as taking advantage of that information.
Audio and Voice Emotion AI
Companies such as Behavioral Signals and Cogito are working on an extraordinary kind of technology for call centres that helps understand how people feel by listening to their voices. This tech can give immediate tips to customer service reps on handling calls better or even help match callers with the best agent for their needs. Sometimes, this voice emotion AI can figure out what people are talking about and how they feel based on how they speak.
HOW DOES AUDIO AND VOICE EMOTION AI WORK?

Skyler Place talks about how, in the past, a lot of focus was on understanding what people say through natural language processing (NLP) and figuring out if their words are positive or negative. But there’s more to conversation than just words. There are “honest signals” – things like how energetic someone’s voice is, the pauses they take, and how their tone goes up and down. These clues help us understand what someone is trying to say, their intentions, and how they feel without having to spell it out.
Now, for the first time, there’s a significant change happening. We’re beginning to combine the traditional way of analyzing words with these honest signals. This combo is a big step forward. It will help us understand and improve how emotions are shared and understood in conversations.
For example, call centres look at around 200 different clues to figure out what’s going on in a conversation. It’s not just about recognizing emotions; it’s about understanding the actions that show those emotions. This way, it’s possible to know how someone feels and to guide the conversation in a way that can change or influence those feelings for the better.
HOW DO CULTURAL DIFFERENCES AFFECT VOICE EMOTION AI?

Rana Gujral talks about how tricky it can be to tell the difference between someone being excited or angry just by their voice since both emotions can sound similar, like having a high pitch. But our brains are good at catching slight differences that tell them apart. He mentions that if you’re watching a movie in a language you don’t understand, you can still guess if a character is mad or excited by their tone. The challenge for technology is figuring out how to understand these subtle differences, too.
They discovered that by adjusting their system to understand the usual way people speak in different languages, they could accurately identify emotions based on small changes in how someone talks. They need a bit of data, between 10 to 50 hours, to get it right for each new language.
The other person, Place, says this is very important because it helps avoid mistakes in their technology that could be caused by needing to understand the context or background of the speaker. They work hard to ensure their system can understand differences in how people speak across different languages and regions, like someone from New York versus the Deep South, and what might be considered a “good” speaking pace or tone in those places.
They handle this by:
- Carefully choose and analyze the data from different places and types of people.
- Having diverse groups of people listen to and evaluate speech samples to avoid bias.
- They are testing their system with a large number of calls from different cultures and use cases to ensure it works well for everyone.
This approach ensures the technology works pretty effectively for all users.
HOW DOES VOICE EMOTION AI DEAL WITH COMPLEXITY?
Gujral mentions that understanding feelings in what people say or write can be pretty tricky. Especially with things like sarcasm, it’s hard for any computer system to get it entirely right. But, for the most part, these systems are good at picking up basic feelings like anger, happiness, sadness, and frustration when someone is just feeling okay.
They also try to figure out if what you’re saying is positive, if your voice sounds different (like more excited or upset), and how you’re acting, like if you’re being polite, really into the conversation, or annoyed.
Plus, they can use unique data to check on things like how well a conversation went or how good someone is at their job. They look for many different clues, and while they’re really good at spotting some feelings, like being super sure when someone’s angry, there are other times they might get it wrong.
HOW CAN VOICE EMOTION AI BE ABUSED?
Gujral, referring to a 2017 study by Yale University’s Professor Michael Krauss, mentioned that people are really good at hiding their emotions when they make facial expressions. Because of this, Gujral thinks using facial expressions to figure out emotions for AI technology can be tricky. If the AI gets it wrong, it could lead to bad outcomes.
Gujral believes there are specific rules we should follow when using this kind of technology. First, people should know and agree to use it, which is all about respecting their privacy and giving them a choice. Then, there are ethical concerns to consider.
For example, Gujral shared a story about turning down a project from a defence company that wanted to use their tech for immigration purposes. The idea was to help make decisions that could seriously change people’s lives, like visa issues or immigration policies. Gujral didn’t want their technology used because the stakes were too high.
WHAT ARE THE CHALLENGES FOR VOICE EMOTION AI?
Gujral says having good-quality data is super important, especially in places like call centres that record calls with good equipment, so there’s little background noise. But this year, there were way more calls than usual, and many were hard to understand because many people were working from home.
Place talks about a tricky tech problem called “synchrony of signals.” This is about making sure different system parts work smoothly together without delay, especially when they’re giving real-time advice. They’ve worked hard to make their software respond quickly.
Still, it’s been challenging to mix the analysis of voice tones (nonverbal signals) with understanding spoken words (natural language processing) because analyzing words takes more time. Figuring out how to blend these two types of information quickly and accurately is a big puzzle for designing the product and ensuring the data flows correctly behind the scenes.
Video and Multimodal Emotion AI
When Emotion AI looks at videos, it doesn’t just see how people’s faces move; it can also notice how they walk or stand to pick up on hidden feelings. Plus, it can use unique cameras to watch where and how long someone’s eyes move or focus on something, like when looking at an ad or surfing a website.
This eye-tracking can show precisely what catches someone’s attention by creating a “heat map” – like a map that shows where the hot spots are based on where people look the most. In some really cool cases, it can even figure out someone’s breathing and heartbeat from a distance, without needing to touch them, just by using the camera.
HOW DOES VIDEO AND MULTIMODAL EMOTION AI WORK?

Daniel McDuff explains that we can now use cameras to understand how people feel because of the fantastic technological improvements over the last 20 years. These cameras are really good at capturing clear images with little background noise. Even with a primary method of analyzing the image, you can look at the colour changes in someone’s skin to figure out their heartbeat and if they’re breathing, as long as they’re not moving too much.
However, when people start moving around, the lighting changes, or because everyone looks different (like different skin colours or having facial hair), things get trickier. That’s where advanced technology called deep learning comes in. It’s brilliant at handling these kinds of problems. The camera might be able to catch the primary signals of what’s happening, but deep learning helps sort through all the extra stuff that doesn’t matter (like changes in lighting or movement) to accurately understand these signals.
HOW DO CULTURAL DIFFERENCES AFFECT VIDEO EMOTION AI?
McDuff explains that people from different cultures can show their emotions differently. Both extensive studies with lots of images and psychology research have found this, whether people are just talking about how they feel or watching and noting it down. But, these cultural differences are usually more minor than the differences between individuals, even those from the same place or family.
For example, someone might be way more outward with their feelings than their sibling, even though they grew up together.
Understanding how cultures differ is definitely excellent and essential for science and psychology. But when analyzing data and figuring out emotions, these cultural differences are often just a tiny part of what makes people unique. Other things, like the situation, a person’s gender, and life experiences, play a more significant role in how they show emotions. How you’re raised, and the behaviour of those around you can shape your expression.
While studying cultural differences is fascinating, the social setting usually matters a lot more in practical situations. If we consider the context, we can make better comparisons between cultures.
For instance, comparing someone singing karaoke in Japan to someone in an office in the US isn’t fair because the situations are entirely different. In the proper context, differences in how people express themselves across cultures can become more apparent.
HOW CAN INDIVIDUALS USE VIDEO EMOTION AI?
McDuff explains that when companies often want to see if people like an ad, they look at how a group reacts, like how much they smile, rather than just one person. They usually watch a bunch of people, maybe 30 or 40, and then average everything.
Marketers like this kind of information and might mix it with other types of feedback. This method doesn’t dive deep into what each person feels inside; it’s more about counting smiles or other visible reactions to measure how the group thinks about something.
However, when it comes to health, things get more personal. You might be trying to see if a behaviour is a sign of something bigger, like a disease symptom or how medicine works. For example, if you’re looking at someone with Parkinson’s disease and how a medicine that stops shaking works over time, you have to focus on that person because everyone’s different. Group data could be more helpful here because you aim to help an individual, not an average of many people.
McDuff is really into projects where you need to customize the approach. For example, if you’re tracking how someone’s heart rate changes, you need to know what’s normal for them because what’s unusual for one person could be just fine for another. While knowing what’s typical for many people can be interesting, more is needed to make a difference individually.
HOW FAR ARE WE FROM MEANINGFUL, POSITIVE IMPACT?
McDuff points out that gadgets like Fitbit can already show how stressed you might be by looking at your heart rate changes over time. Whether this info comes from a wearable device, a camera, or something else doesn’t really matter—it’s all about the type of sensor used.
However, The real challenge is making this data useful to us.
For example, if your device counts your steps and you see you’ve only walked a little, it can simply suggest you walk more the next day. But figuring out what to do next isn’t as straightforward when it comes to stress.
He believes we’re still a bit away from turning the tracking of things like stress into something beneficial. It could take years because dealing with complex issues like stress requires personalized solutions. The main goal is to figure out how to use all this tracking data—not just to collect it since we already have plenty of data—but to turn it into insights that actually make the devices more helpful for people.
HOW CAN VIDEO EMOTION AI BE ABUSED?
McDuff emphasizes that before we use technology to solve a problem, we must ensure it helps in that situation. The first step is to look for a solid proof that the technology will make a positive difference.
He also discusses the importance of considering who the technology is being used by. Often, AI is used when people are already in a tough spot, like job seekers or students. Companies and schools have more power in these situations because they make big decisions like hiring or grading. It’s crucial to consider whether the technology is just making things easier for those who already have the power, possibly at the expense of making things less fair or confusing for others.
For example, there’s a big issue with schools using software to watch students during tests to prevent cheating. These systems might not catch all the subtle things that a human would, and wrongly accusing someone of cheating can have serious consequences. McDuff suggests that there are better approaches than throwing machine learning at problems like this without thinking it through.